Parquet with Athena VS RedshiftSpark Redshift saving into s3 as ParquetRedshift COPY command for Parquet format with Snappy compressionSpark & Parquet Query PerformanceIs there a data architecture for efficient joins in Spark (a la RedShift)?Apahce Spark on Redshift vs Apache Spark on HIVE EMRWhich would be a quicker (and better) tool for querying data stored in the Parquet format - Spark SQL, Athena or ElasticSearch?Error while Connecting PySpark to AWS RedshiftAthena/Hive timestamp in parquet files written by sparkSpark 2.3.1 AWS EMR not returning data for some columns yet works in Athena/Presto and SpectrumQuery Cassandra UDT via Spark SQL
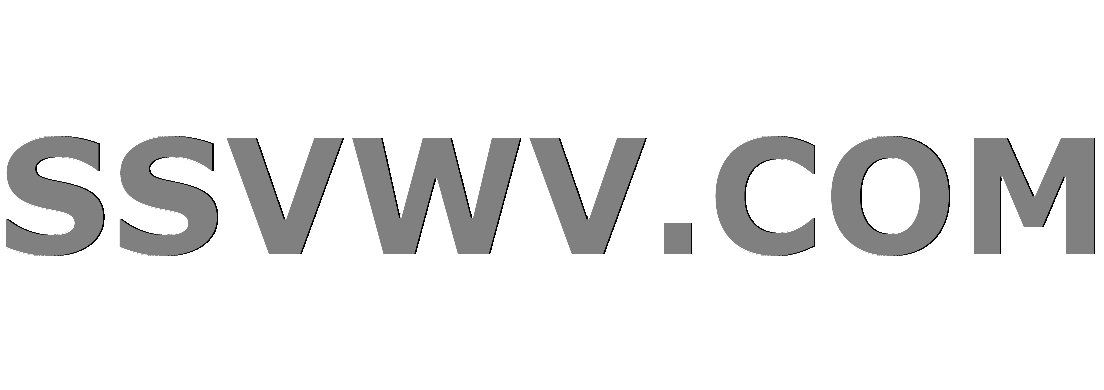
Multi tool use
What defenses are there against being summoned by the Gate spell?
Why can't I see bouncing of a switch on an oscilloscope?
Accidentally leaked the solution to an assignment, what to do now? (I'm the prof)
Is it important to consider tone, melody, and musical form while writing a song?
Why not use SQL instead of GraphQL?
Arthur Somervell: 1000 Exercises - Meaning of this notation
How do we improve the relationship with a client software team that performs poorly and is becoming less collaborative?
If I cast Expeditious Retreat, can I Dash as a bonus action on the same turn?
What do the dots in this tr command do: tr .............A-Z A-ZA-Z <<< "JVPQBOV" (with 13 dots)
How to say job offer in Mandarin/Cantonese?
What's the point of deactivating Num Lock on login screens?
What typically incentivizes a professor to change jobs to a lower ranking university?
How can I make my BBEG immortal short of making them a Lich or Vampire?
How could an uplifted falcon's brain work?
Show that if two triangles built on parallel lines, with equal bases have the same perimeter only if they are congruent.
What's the output of a record cartridge playing an out-of-speed record
Can I make popcorn with any corn?
Today is the Center
How do I create uniquely male characters?
Why did the Germans forbid the possession of pet pigeons in Rostov-on-Don in 1941?
strToHex ( string to its hex representation as string)
Fencing style for blades that can attack from a distance
Can divisibility rules for digits be generalized to sum of digits
Have astronauts in space suits ever taken selfies? If so, how?
Parquet with Athena VS Redshift
Spark Redshift saving into s3 as ParquetRedshift COPY command for Parquet format with Snappy compressionSpark & Parquet Query PerformanceIs there a data architecture for efficient joins in Spark (a la RedShift)?Apahce Spark on Redshift vs Apache Spark on HIVE EMRWhich would be a quicker (and better) tool for querying data stored in the Parquet format - Spark SQL, Athena or ElasticSearch?Error while Connecting PySpark to AWS RedshiftAthena/Hive timestamp in parquet files written by sparkSpark 2.3.1 AWS EMR not returning data for some columns yet works in Athena/Presto and SpectrumQuery Cassandra UDT via Spark SQL
.everyoneloves__top-leaderboard:empty,.everyoneloves__mid-leaderboard:empty,.everyoneloves__bot-mid-leaderboard:empty height:90px;width:728px;box-sizing:border-box;
I hope someone out there can help me with this issue. I am currently working on a data pipeline project, my current dilemma is whether to use parquet with Athena or storing it to Redshift
2 Scenarios:
First,
EVENTS --> STORE IT IN S3 AS JSON.GZ --> USE SPARK(EMR) TO CONVERT TO PARQUET --> STORE PARQUET BACK INTO S3 --> ATHENA FOR QUERY --> VIZ
Second,
EVENTS --> STORE IT IN S3 --> USE SPARK(EMR) TO STORE DATA INTO REDSHIFT
Issues with this scenario:
- Spark JDBC with Redshift is slow
- Spark-Redshift repo by data bricks have a fail build and was updated 2 years ago
I am unable to find useful information on which method is better. Should I even use Redshift or is parquet good enough?
Also it would be great if someone could tell me if there are any other methods for connecting spark with Redshift because there's only 2 solution that I saw online - JDBC and Spark-Reshift(Databricks)
P.S. the pricing model is not a concern to me also I'm dealing with millions of events data.
apache-spark amazon-s3 amazon-redshift parquet
add a comment |
I hope someone out there can help me with this issue. I am currently working on a data pipeline project, my current dilemma is whether to use parquet with Athena or storing it to Redshift
2 Scenarios:
First,
EVENTS --> STORE IT IN S3 AS JSON.GZ --> USE SPARK(EMR) TO CONVERT TO PARQUET --> STORE PARQUET BACK INTO S3 --> ATHENA FOR QUERY --> VIZ
Second,
EVENTS --> STORE IT IN S3 --> USE SPARK(EMR) TO STORE DATA INTO REDSHIFT
Issues with this scenario:
- Spark JDBC with Redshift is slow
- Spark-Redshift repo by data bricks have a fail build and was updated 2 years ago
I am unable to find useful information on which method is better. Should I even use Redshift or is parquet good enough?
Also it would be great if someone could tell me if there are any other methods for connecting spark with Redshift because there's only 2 solution that I saw online - JDBC and Spark-Reshift(Databricks)
P.S. the pricing model is not a concern to me also I'm dealing with millions of events data.
apache-spark amazon-s3 amazon-redshift parquet
add a comment |
I hope someone out there can help me with this issue. I am currently working on a data pipeline project, my current dilemma is whether to use parquet with Athena or storing it to Redshift
2 Scenarios:
First,
EVENTS --> STORE IT IN S3 AS JSON.GZ --> USE SPARK(EMR) TO CONVERT TO PARQUET --> STORE PARQUET BACK INTO S3 --> ATHENA FOR QUERY --> VIZ
Second,
EVENTS --> STORE IT IN S3 --> USE SPARK(EMR) TO STORE DATA INTO REDSHIFT
Issues with this scenario:
- Spark JDBC with Redshift is slow
- Spark-Redshift repo by data bricks have a fail build and was updated 2 years ago
I am unable to find useful information on which method is better. Should I even use Redshift or is parquet good enough?
Also it would be great if someone could tell me if there are any other methods for connecting spark with Redshift because there's only 2 solution that I saw online - JDBC and Spark-Reshift(Databricks)
P.S. the pricing model is not a concern to me also I'm dealing with millions of events data.
apache-spark amazon-s3 amazon-redshift parquet
I hope someone out there can help me with this issue. I am currently working on a data pipeline project, my current dilemma is whether to use parquet with Athena or storing it to Redshift
2 Scenarios:
First,
EVENTS --> STORE IT IN S3 AS JSON.GZ --> USE SPARK(EMR) TO CONVERT TO PARQUET --> STORE PARQUET BACK INTO S3 --> ATHENA FOR QUERY --> VIZ
Second,
EVENTS --> STORE IT IN S3 --> USE SPARK(EMR) TO STORE DATA INTO REDSHIFT
Issues with this scenario:
- Spark JDBC with Redshift is slow
- Spark-Redshift repo by data bricks have a fail build and was updated 2 years ago
I am unable to find useful information on which method is better. Should I even use Redshift or is parquet good enough?
Also it would be great if someone could tell me if there are any other methods for connecting spark with Redshift because there's only 2 solution that I saw online - JDBC and Spark-Reshift(Databricks)
P.S. the pricing model is not a concern to me also I'm dealing with millions of events data.
apache-spark amazon-s3 amazon-redshift parquet
apache-spark amazon-s3 amazon-redshift parquet
edited Mar 8 at 7:34
Red Boy
2,26621124
2,26621124
asked Mar 8 at 4:15


Louis WongLouis Wong
93
93
add a comment |
add a comment |
2 Answers
2
active
oldest
votes
Here are some ideas / recommendations
- Don't use JDBC.
- Spark-Redshift works fine but is a complex solution.
- You don't have to use spark to convert to parquet, there is also the option of using hive.
see
https://docs.aws.amazon.com/athena/latest/ug/convert-to-columnar.html - Athena is great when used against parquet, so you don't need to use
Redshift at all - If you want to use Redshift, then use Redshift spectrum to set up a
view against your parquet tables, then if necessary a CTAS within
Redshift to bring the data in if you need to. - AWS Glue Crawler can be a great way to create the metadata needed to
map the parquet in to Athena and Redshift Spectrum.
My proposed architecture:
EVENTS --> STORE IT IN S3 --> HIVE to convert to parquet --> Use directly in Athena
and/or
EVENTS --> STORE IT IN S3 --> HIVE to convert to parquet --> Use directly in Redshift using Redshift Spectrum
You MAY NOT need to convert to parquet, if you use the right partitioning structure (s3 folders) and gzip the data then Athena/spectrum then performance can be good enough without the complexity of conversion to parquet. This is dependent on your use case (volumes of data and types of query that you need to run).
add a comment |
Which one to use depends on your data and access patterns. Athena directly uses S3 key structure to limit the amount of data to be scanned. Let's assume you have event type and time in events. The S3 keys could be e.g. yyyy/MM/dd/type/*
or type/yyyy/MM/dd/*
. The former key structure allows you to limit the amount of data to be scanned by date or date and type but not type alone. If you wanted to search only by type x
but don't know the date, it would require a full bucket scan. The latter key schema would be the other way around. If you mostly need to access the data just one way (e.g. by time), Athena might be a good choice.
On the other hand, Redshift is a PostgreSQL based data warehouse which is much more complicated and flexible than Athena. The data partitioning plays a big role in terms of performance, but schema can be designed in many ways to suit your use-case. In my experience the best way to load data to Redshift is first to store it to S3 and then use COPY https://docs.aws.amazon.com/redshift/latest/dg/r_COPY.html . It is multiple magnitudes faster than JDBC which I found only good for testing with small amounts of data. This is also how Kinesis Firehose loads data into Redshift. If you don't want to implement S3 copying yourself, Firehose provides an alternative for that.
add a comment |
Your Answer
StackExchange.ifUsing("editor", function ()
StackExchange.using("externalEditor", function ()
StackExchange.using("snippets", function ()
StackExchange.snippets.init();
);
);
, "code-snippets");
StackExchange.ready(function()
var channelOptions =
tags: "".split(" "),
id: "1"
;
initTagRenderer("".split(" "), "".split(" "), channelOptions);
StackExchange.using("externalEditor", function()
// Have to fire editor after snippets, if snippets enabled
if (StackExchange.settings.snippets.snippetsEnabled)
StackExchange.using("snippets", function()
createEditor();
);
else
createEditor();
);
function createEditor()
StackExchange.prepareEditor(
heartbeatType: 'answer',
autoActivateHeartbeat: false,
convertImagesToLinks: true,
noModals: true,
showLowRepImageUploadWarning: true,
reputationToPostImages: 10,
bindNavPrevention: true,
postfix: "",
imageUploader:
brandingHtml: "Powered by u003ca class="icon-imgur-white" href="https://imgur.com/"u003eu003c/au003e",
contentPolicyHtml: "User contributions licensed under u003ca href="https://creativecommons.org/licenses/by-sa/3.0/"u003ecc by-sa 3.0 with attribution requiredu003c/au003e u003ca href="https://stackoverflow.com/legal/content-policy"u003e(content policy)u003c/au003e",
allowUrls: true
,
onDemand: true,
discardSelector: ".discard-answer"
,immediatelyShowMarkdownHelp:true
);
);
Sign up or log in
StackExchange.ready(function ()
StackExchange.helpers.onClickDraftSave('#login-link');
);
Sign up using Google
Sign up using Facebook
Sign up using Email and Password
Post as a guest
Required, but never shown
StackExchange.ready(
function ()
StackExchange.openid.initPostLogin('.new-post-login', 'https%3a%2f%2fstackoverflow.com%2fquestions%2f55056640%2fparquet-with-athena-vs-redshift%23new-answer', 'question_page');
);
Post as a guest
Required, but never shown
2 Answers
2
active
oldest
votes
2 Answers
2
active
oldest
votes
active
oldest
votes
active
oldest
votes
Here are some ideas / recommendations
- Don't use JDBC.
- Spark-Redshift works fine but is a complex solution.
- You don't have to use spark to convert to parquet, there is also the option of using hive.
see
https://docs.aws.amazon.com/athena/latest/ug/convert-to-columnar.html - Athena is great when used against parquet, so you don't need to use
Redshift at all - If you want to use Redshift, then use Redshift spectrum to set up a
view against your parquet tables, then if necessary a CTAS within
Redshift to bring the data in if you need to. - AWS Glue Crawler can be a great way to create the metadata needed to
map the parquet in to Athena and Redshift Spectrum.
My proposed architecture:
EVENTS --> STORE IT IN S3 --> HIVE to convert to parquet --> Use directly in Athena
and/or
EVENTS --> STORE IT IN S3 --> HIVE to convert to parquet --> Use directly in Redshift using Redshift Spectrum
You MAY NOT need to convert to parquet, if you use the right partitioning structure (s3 folders) and gzip the data then Athena/spectrum then performance can be good enough without the complexity of conversion to parquet. This is dependent on your use case (volumes of data and types of query that you need to run).
add a comment |
Here are some ideas / recommendations
- Don't use JDBC.
- Spark-Redshift works fine but is a complex solution.
- You don't have to use spark to convert to parquet, there is also the option of using hive.
see
https://docs.aws.amazon.com/athena/latest/ug/convert-to-columnar.html - Athena is great when used against parquet, so you don't need to use
Redshift at all - If you want to use Redshift, then use Redshift spectrum to set up a
view against your parquet tables, then if necessary a CTAS within
Redshift to bring the data in if you need to. - AWS Glue Crawler can be a great way to create the metadata needed to
map the parquet in to Athena and Redshift Spectrum.
My proposed architecture:
EVENTS --> STORE IT IN S3 --> HIVE to convert to parquet --> Use directly in Athena
and/or
EVENTS --> STORE IT IN S3 --> HIVE to convert to parquet --> Use directly in Redshift using Redshift Spectrum
You MAY NOT need to convert to parquet, if you use the right partitioning structure (s3 folders) and gzip the data then Athena/spectrum then performance can be good enough without the complexity of conversion to parquet. This is dependent on your use case (volumes of data and types of query that you need to run).
add a comment |
Here are some ideas / recommendations
- Don't use JDBC.
- Spark-Redshift works fine but is a complex solution.
- You don't have to use spark to convert to parquet, there is also the option of using hive.
see
https://docs.aws.amazon.com/athena/latest/ug/convert-to-columnar.html - Athena is great when used against parquet, so you don't need to use
Redshift at all - If you want to use Redshift, then use Redshift spectrum to set up a
view against your parquet tables, then if necessary a CTAS within
Redshift to bring the data in if you need to. - AWS Glue Crawler can be a great way to create the metadata needed to
map the parquet in to Athena and Redshift Spectrum.
My proposed architecture:
EVENTS --> STORE IT IN S3 --> HIVE to convert to parquet --> Use directly in Athena
and/or
EVENTS --> STORE IT IN S3 --> HIVE to convert to parquet --> Use directly in Redshift using Redshift Spectrum
You MAY NOT need to convert to parquet, if you use the right partitioning structure (s3 folders) and gzip the data then Athena/spectrum then performance can be good enough without the complexity of conversion to parquet. This is dependent on your use case (volumes of data and types of query that you need to run).
Here are some ideas / recommendations
- Don't use JDBC.
- Spark-Redshift works fine but is a complex solution.
- You don't have to use spark to convert to parquet, there is also the option of using hive.
see
https://docs.aws.amazon.com/athena/latest/ug/convert-to-columnar.html - Athena is great when used against parquet, so you don't need to use
Redshift at all - If you want to use Redshift, then use Redshift spectrum to set up a
view against your parquet tables, then if necessary a CTAS within
Redshift to bring the data in if you need to. - AWS Glue Crawler can be a great way to create the metadata needed to
map the parquet in to Athena and Redshift Spectrum.
My proposed architecture:
EVENTS --> STORE IT IN S3 --> HIVE to convert to parquet --> Use directly in Athena
and/or
EVENTS --> STORE IT IN S3 --> HIVE to convert to parquet --> Use directly in Redshift using Redshift Spectrum
You MAY NOT need to convert to parquet, if you use the right partitioning structure (s3 folders) and gzip the data then Athena/spectrum then performance can be good enough without the complexity of conversion to parquet. This is dependent on your use case (volumes of data and types of query that you need to run).
answered Mar 8 at 8:06
Jon ScottJon Scott
2,107718
2,107718
add a comment |
add a comment |
Which one to use depends on your data and access patterns. Athena directly uses S3 key structure to limit the amount of data to be scanned. Let's assume you have event type and time in events. The S3 keys could be e.g. yyyy/MM/dd/type/*
or type/yyyy/MM/dd/*
. The former key structure allows you to limit the amount of data to be scanned by date or date and type but not type alone. If you wanted to search only by type x
but don't know the date, it would require a full bucket scan. The latter key schema would be the other way around. If you mostly need to access the data just one way (e.g. by time), Athena might be a good choice.
On the other hand, Redshift is a PostgreSQL based data warehouse which is much more complicated and flexible than Athena. The data partitioning plays a big role in terms of performance, but schema can be designed in many ways to suit your use-case. In my experience the best way to load data to Redshift is first to store it to S3 and then use COPY https://docs.aws.amazon.com/redshift/latest/dg/r_COPY.html . It is multiple magnitudes faster than JDBC which I found only good for testing with small amounts of data. This is also how Kinesis Firehose loads data into Redshift. If you don't want to implement S3 copying yourself, Firehose provides an alternative for that.
add a comment |
Which one to use depends on your data and access patterns. Athena directly uses S3 key structure to limit the amount of data to be scanned. Let's assume you have event type and time in events. The S3 keys could be e.g. yyyy/MM/dd/type/*
or type/yyyy/MM/dd/*
. The former key structure allows you to limit the amount of data to be scanned by date or date and type but not type alone. If you wanted to search only by type x
but don't know the date, it would require a full bucket scan. The latter key schema would be the other way around. If you mostly need to access the data just one way (e.g. by time), Athena might be a good choice.
On the other hand, Redshift is a PostgreSQL based data warehouse which is much more complicated and flexible than Athena. The data partitioning plays a big role in terms of performance, but schema can be designed in many ways to suit your use-case. In my experience the best way to load data to Redshift is first to store it to S3 and then use COPY https://docs.aws.amazon.com/redshift/latest/dg/r_COPY.html . It is multiple magnitudes faster than JDBC which I found only good for testing with small amounts of data. This is also how Kinesis Firehose loads data into Redshift. If you don't want to implement S3 copying yourself, Firehose provides an alternative for that.
add a comment |
Which one to use depends on your data and access patterns. Athena directly uses S3 key structure to limit the amount of data to be scanned. Let's assume you have event type and time in events. The S3 keys could be e.g. yyyy/MM/dd/type/*
or type/yyyy/MM/dd/*
. The former key structure allows you to limit the amount of data to be scanned by date or date and type but not type alone. If you wanted to search only by type x
but don't know the date, it would require a full bucket scan. The latter key schema would be the other way around. If you mostly need to access the data just one way (e.g. by time), Athena might be a good choice.
On the other hand, Redshift is a PostgreSQL based data warehouse which is much more complicated and flexible than Athena. The data partitioning plays a big role in terms of performance, but schema can be designed in many ways to suit your use-case. In my experience the best way to load data to Redshift is first to store it to S3 and then use COPY https://docs.aws.amazon.com/redshift/latest/dg/r_COPY.html . It is multiple magnitudes faster than JDBC which I found only good for testing with small amounts of data. This is also how Kinesis Firehose loads data into Redshift. If you don't want to implement S3 copying yourself, Firehose provides an alternative for that.
Which one to use depends on your data and access patterns. Athena directly uses S3 key structure to limit the amount of data to be scanned. Let's assume you have event type and time in events. The S3 keys could be e.g. yyyy/MM/dd/type/*
or type/yyyy/MM/dd/*
. The former key structure allows you to limit the amount of data to be scanned by date or date and type but not type alone. If you wanted to search only by type x
but don't know the date, it would require a full bucket scan. The latter key schema would be the other way around. If you mostly need to access the data just one way (e.g. by time), Athena might be a good choice.
On the other hand, Redshift is a PostgreSQL based data warehouse which is much more complicated and flexible than Athena. The data partitioning plays a big role in terms of performance, but schema can be designed in many ways to suit your use-case. In my experience the best way to load data to Redshift is first to store it to S3 and then use COPY https://docs.aws.amazon.com/redshift/latest/dg/r_COPY.html . It is multiple magnitudes faster than JDBC which I found only good for testing with small amounts of data. This is also how Kinesis Firehose loads data into Redshift. If you don't want to implement S3 copying yourself, Firehose provides an alternative for that.
answered Mar 8 at 8:07


ollik1ollik1
67819
67819
add a comment |
add a comment |
Thanks for contributing an answer to Stack Overflow!
- Please be sure to answer the question. Provide details and share your research!
But avoid …
- Asking for help, clarification, or responding to other answers.
- Making statements based on opinion; back them up with references or personal experience.
To learn more, see our tips on writing great answers.
Sign up or log in
StackExchange.ready(function ()
StackExchange.helpers.onClickDraftSave('#login-link');
);
Sign up using Google
Sign up using Facebook
Sign up using Email and Password
Post as a guest
Required, but never shown
StackExchange.ready(
function ()
StackExchange.openid.initPostLogin('.new-post-login', 'https%3a%2f%2fstackoverflow.com%2fquestions%2f55056640%2fparquet-with-athena-vs-redshift%23new-answer', 'question_page');
);
Post as a guest
Required, but never shown
Sign up or log in
StackExchange.ready(function ()
StackExchange.helpers.onClickDraftSave('#login-link');
);
Sign up using Google
Sign up using Facebook
Sign up using Email and Password
Post as a guest
Required, but never shown
Sign up or log in
StackExchange.ready(function ()
StackExchange.helpers.onClickDraftSave('#login-link');
);
Sign up using Google
Sign up using Facebook
Sign up using Email and Password
Post as a guest
Required, but never shown
Sign up or log in
StackExchange.ready(function ()
StackExchange.helpers.onClickDraftSave('#login-link');
);
Sign up using Google
Sign up using Facebook
Sign up using Email and Password
Sign up using Google
Sign up using Facebook
Sign up using Email and Password
Post as a guest
Required, but never shown
Required, but never shown
Required, but never shown
Required, but never shown
Required, but never shown
Required, but never shown
Required, but never shown
Required, but never shown
Required, but never shown
p czOaG3uV5X6,4Zz Tly XA6sV0LnlQmrYIwPwcybIXxoDCSRtE9,0hxUqsLJGp zsszm4gwWlJhj6mZUrJcLzJ6