Identifying and removing invalid time steps in a pandas time series Announcing the arrival of Valued Associate #679: Cesar Manara Planned maintenance scheduled April 23, 2019 at 23:30 UTC (7:30pm US/Eastern) Data science time! April 2019 and salary with experience The Ask Question Wizard is Live!Remove rows with duplicate indices (Pandas DataFrame and TimeSeries)Selecting a row of pandas series/dataframe by integer indexCombining two Series into a DataFrame in pandasPretty-print an entire Pandas Series / DataFramePandas conditional creation of a series/dataframe columnTime-series boxplot in pandasTime series Analysis in Rhow to sort from greatest to least in a .csv file in pythonread data table from .txt file and parse it to variablesorting instance of dict_items/sorting dictionary by value
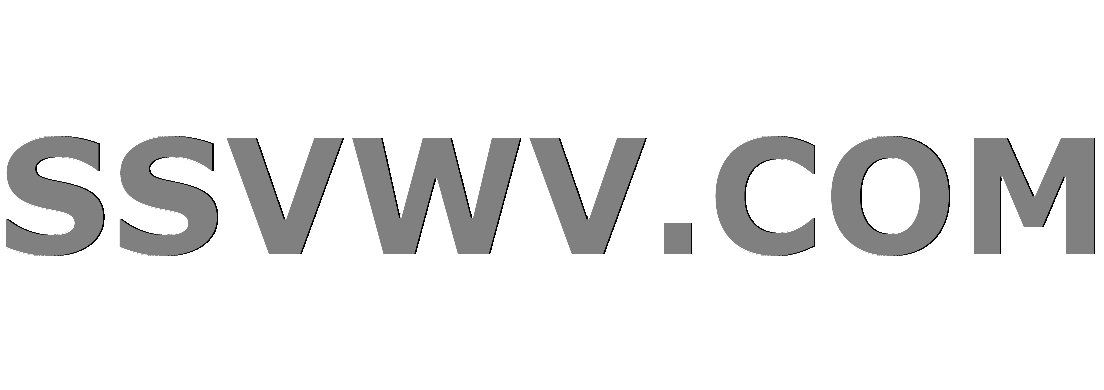
Multi tool use
Will I be more secure with my own router behind my ISP's router?
Putting Ant-Man on house arrest
Short story about an alien named Ushtu(?) coming from a future Earth, when ours was destroyed by a nuclear explosion
Why does BitLocker not use RSA?
Is there a verb for listening stealthily?
How to mute a string and play another at the same time
Is Bran literally the world's memory?
How can I introduce the names of fantasy creatures to the reader?
Does Prince Arnaud cause someone holding the Princess to lose?
Why does my GNOME settings mention "Moto C Plus"?
What is the definining line between a helicopter and a drone a person can ride in?
Why these surprising proportionalities of integrals involving odd zeta values?
When does Bran Stark remember Jamie pushing him?
Is there a way to convert Wolfram Language expression to string?
Can gravitational waves pass through a black hole?
Why are two-digit numbers in Jonathan Swift's "Gulliver's Travels" (1726) written in "German style"?
Network questions
Does traveling In The United States require a passport or can I use my green card if not a US citizen?
Does the Pact of the Blade warlock feature allow me to customize the properties of the pact weapon I create?
What is the evidence that custom checks in Northern Ireland are going to result in violence?
Etymology of 見舞い
Protagonist's race is hidden - should I reveal it?
Are there any AGPL-style licences that require source code modifications to be public?
What is the difference between 准时 and 按时?
Identifying and removing invalid time steps in a pandas time series
Announcing the arrival of Valued Associate #679: Cesar Manara
Planned maintenance scheduled April 23, 2019 at 23:30 UTC (7:30pm US/Eastern)
Data science time! April 2019 and salary with experience
The Ask Question Wizard is Live!Remove rows with duplicate indices (Pandas DataFrame and TimeSeries)Selecting a row of pandas series/dataframe by integer indexCombining two Series into a DataFrame in pandasPretty-print an entire Pandas Series / DataFramePandas conditional creation of a series/dataframe columnTime-series boxplot in pandasTime series Analysis in Rhow to sort from greatest to least in a .csv file in pythonread data table from .txt file and parse it to variablesorting instance of dict_items/sorting dictionary by value
.everyoneloves__top-leaderboard:empty,.everyoneloves__mid-leaderboard:empty,.everyoneloves__bot-mid-leaderboard:empty height:90px;width:728px;box-sizing:border-box;
I looked for an answer for this for a while but haven't been able to find anything, so forgive me if this question has been asked before...
I have some 6-hourly timeseries data future
of future temperature projections for the years 2031-2050. Upon looking at the data, I notice that there is some faulty timedeltas in the dataset starting at future.iloc[234]
:
future.iloc[220:281]
time Temp
220 2031-03-28 00:00:00 68.276657
221 2031-03-28 06:00:00 68.270706
222 2031-03-28 12:00:00 68.264748
223 2031-03-28 18:00:00 68.258781
224 2031-03-29 00:00:00 68.252808
225 2031-03-29 06:00:00 68.246849
226 2031-03-29 12:00:00 68.240883
227 2031-03-29 18:00:00 68.234909
228 2031-03-30 00:00:00 68.228943
229 2031-03-30 06:00:00 68.222984
230 2031-03-30 12:00:00 68.217010
231 2031-03-30 18:00:00 68.211052
232 2031-03-31 00:00:00 68.205093
233 2031-03-31 06:00:00 68.199120
234 2031-03-31 12:00:00 68.193153
235 2031-02-26 00:00:00 68.187195
236 2031-02-26 06:00:00 68.181236
237 2031-02-26 12:00:00 68.175270
238 2031-02-26 18:00:00 68.169304
239 2031-02-27 00:00:00 68.163322
240 2031-02-27 06:00:00 68.169304
....
369 2031-03-31 12:00:00 68.193153
370 2031-03-31 18:00:00 68.258781
371 2031-04-01 00:00:00 67.950096
372 2031-04-01 06:00:00 67.949493
373 2031-04-01 12:00:00 67.949539
374 2031-04-01 18:00:00 67.950241
375 2031-04-02 00:00:00 67.951591
376 2031-04-02 06:00:00 67.953590
377 2031-04-02 12:00:00 67.955589
378 2031-04-02 18:00:00 67.957596
379 2031-04-03 00:00:00 67.959595
380 2031-04-03 06:00:00 67.961601
The dataset continues with the correct timedelta after this blip, but seems to repeat just over a whole month of data (i.e. future.iloc[370] = 2031-03-31 18:00:00
which should be the next timestep after future.iloc[234]
, and continues with valid data from this point on).I know that the data (other than the repeated month) is valid, so I need to try an salvage the data if I can. I have a number of these datasets, so I now fear that they may have faulty time steps in them as well.
My goal is to check for an inconsistent timedelta between two points, and either remove the rows with the invalid timedeltas:
time Temp
220 2031-03-28 00:00:00 68.276657
221 2031-03-28 06:00:00 68.270706
222 2031-03-28 12:00:00 68.264748
223 2031-03-28 18:00:00 68.258781
224 2031-03-29 00:00:00 68.252808
225 2031-03-29 06:00:00 68.246849
226 2031-03-29 12:00:00 68.240883
227 2031-03-29 18:00:00 68.234909
228 2031-03-30 00:00:00 68.228943
229 2031-03-30 06:00:00 68.222984
230 2031-03-30 12:00:00 68.217010
231 2031-03-30 18:00:00 68.211052
232 2031-03-31 00:00:00 68.205093
233 2031-03-31 06:00:00 68.199120
234 2031-03-31 12:00:00 68.193153
235 2031-03-31 18:00:00 68.258781
236 2031-04-01 00:00:00 67.950096
237 2031-04-01 06:00:00 67.949493
238 2031-04-01 12:00:00 67.949539
239 2031-04-01 18:00:00 67.950241
240 2031-04-02 00:00:00 67.951591
241 2031-04-02 06:00:00 67.953590
242 2031-04-02 12:00:00 67.955589
243 2031-04-02 18:00:00 67.957596
244 2031-04-03 00:00:00 67.959595
245 2031-04-03 06:00:00 67.961601
or null all data that is associated with an invalid timedelta:
time Temp
220 2031-03-28 00:00:00 68.276657
221 2031-03-28 06:00:00 68.270706
222 2031-03-28 12:00:00 68.264748
223 2031-03-28 18:00:00 68.258781
224 2031-03-29 00:00:00 68.252808
225 2031-03-29 06:00:00 68.246849
226 2031-03-29 12:00:00 68.240883
227 2031-03-29 18:00:00 68.234909
228 2031-03-30 00:00:00 68.228943
229 2031-03-30 06:00:00 68.222984
230 2031-03-30 12:00:00 68.217010
231 2031-03-30 18:00:00 68.211052
232 2031-03-31 00:00:00 68.205093
233 2031-03-31 06:00:00 68.199120
234 2031-03-31 12:00:00 68.193153
235 2031-02-26 00:00:00 NaN
236 2031-02-26 06:00:00 NaN
237 2031-02-26 12:00:00 NaN
238 2031-02-26 18:00:00 NaN
239 2031-02-27 00:00:00 NaN
240 2031-02-27 06:00:00 NaN
....
369 2031-03-31 12:00:00 NaN
370 2031-03-31 18:00:00 68.258781
371 2031-04-01 00:00:00 67.950096
372 2031-04-01 06:00:00 67.949493
373 2031-04-01 12:00:00 67.949539
374 2031-04-01 18:00:00 67.950241
375 2031-04-02 00:00:00 67.951591
376 2031-04-02 06:00:00 67.953590
377 2031-04-02 12:00:00 67.955589
378 2031-04-02 18:00:00 67.957596
379 2031-04-03 00:00:00 67.959595
380 2031-04-03 06:00:00 67.961601
The real problem I can't fully wrap my head around is that only future.iloc[235]
is an invalid timedelta. future.iloc[236:270]
are still technically correct 6H timesteps, they have just been offset, which causes the duplication. So to fully remove invalid data, I need to identify both the invalid timedelta as well as the valid timedeltas that create the duplicate data.
I have attempted to create a comparison date range with pd.date_range(start=future.iloc[0].time, end=future.iloc[-1].time, freq='6H')
, and iterate through my rows to find faulty values. However, I have not been able to come up with a solution that will actually identify and remove the faulty rows.
Any ideas on how to do this? I assumed pandas would have some built-in functionality for something like this, but haven't been able to find anything substantial that fits my needs.
Bonus: Every check that I have tried seems to take up to minute to run through about 30,000 rows of data. Does this number of rows warrant this time usage iterate through?
python pandas time-series python-xarray
add a comment |
I looked for an answer for this for a while but haven't been able to find anything, so forgive me if this question has been asked before...
I have some 6-hourly timeseries data future
of future temperature projections for the years 2031-2050. Upon looking at the data, I notice that there is some faulty timedeltas in the dataset starting at future.iloc[234]
:
future.iloc[220:281]
time Temp
220 2031-03-28 00:00:00 68.276657
221 2031-03-28 06:00:00 68.270706
222 2031-03-28 12:00:00 68.264748
223 2031-03-28 18:00:00 68.258781
224 2031-03-29 00:00:00 68.252808
225 2031-03-29 06:00:00 68.246849
226 2031-03-29 12:00:00 68.240883
227 2031-03-29 18:00:00 68.234909
228 2031-03-30 00:00:00 68.228943
229 2031-03-30 06:00:00 68.222984
230 2031-03-30 12:00:00 68.217010
231 2031-03-30 18:00:00 68.211052
232 2031-03-31 00:00:00 68.205093
233 2031-03-31 06:00:00 68.199120
234 2031-03-31 12:00:00 68.193153
235 2031-02-26 00:00:00 68.187195
236 2031-02-26 06:00:00 68.181236
237 2031-02-26 12:00:00 68.175270
238 2031-02-26 18:00:00 68.169304
239 2031-02-27 00:00:00 68.163322
240 2031-02-27 06:00:00 68.169304
....
369 2031-03-31 12:00:00 68.193153
370 2031-03-31 18:00:00 68.258781
371 2031-04-01 00:00:00 67.950096
372 2031-04-01 06:00:00 67.949493
373 2031-04-01 12:00:00 67.949539
374 2031-04-01 18:00:00 67.950241
375 2031-04-02 00:00:00 67.951591
376 2031-04-02 06:00:00 67.953590
377 2031-04-02 12:00:00 67.955589
378 2031-04-02 18:00:00 67.957596
379 2031-04-03 00:00:00 67.959595
380 2031-04-03 06:00:00 67.961601
The dataset continues with the correct timedelta after this blip, but seems to repeat just over a whole month of data (i.e. future.iloc[370] = 2031-03-31 18:00:00
which should be the next timestep after future.iloc[234]
, and continues with valid data from this point on).I know that the data (other than the repeated month) is valid, so I need to try an salvage the data if I can. I have a number of these datasets, so I now fear that they may have faulty time steps in them as well.
My goal is to check for an inconsistent timedelta between two points, and either remove the rows with the invalid timedeltas:
time Temp
220 2031-03-28 00:00:00 68.276657
221 2031-03-28 06:00:00 68.270706
222 2031-03-28 12:00:00 68.264748
223 2031-03-28 18:00:00 68.258781
224 2031-03-29 00:00:00 68.252808
225 2031-03-29 06:00:00 68.246849
226 2031-03-29 12:00:00 68.240883
227 2031-03-29 18:00:00 68.234909
228 2031-03-30 00:00:00 68.228943
229 2031-03-30 06:00:00 68.222984
230 2031-03-30 12:00:00 68.217010
231 2031-03-30 18:00:00 68.211052
232 2031-03-31 00:00:00 68.205093
233 2031-03-31 06:00:00 68.199120
234 2031-03-31 12:00:00 68.193153
235 2031-03-31 18:00:00 68.258781
236 2031-04-01 00:00:00 67.950096
237 2031-04-01 06:00:00 67.949493
238 2031-04-01 12:00:00 67.949539
239 2031-04-01 18:00:00 67.950241
240 2031-04-02 00:00:00 67.951591
241 2031-04-02 06:00:00 67.953590
242 2031-04-02 12:00:00 67.955589
243 2031-04-02 18:00:00 67.957596
244 2031-04-03 00:00:00 67.959595
245 2031-04-03 06:00:00 67.961601
or null all data that is associated with an invalid timedelta:
time Temp
220 2031-03-28 00:00:00 68.276657
221 2031-03-28 06:00:00 68.270706
222 2031-03-28 12:00:00 68.264748
223 2031-03-28 18:00:00 68.258781
224 2031-03-29 00:00:00 68.252808
225 2031-03-29 06:00:00 68.246849
226 2031-03-29 12:00:00 68.240883
227 2031-03-29 18:00:00 68.234909
228 2031-03-30 00:00:00 68.228943
229 2031-03-30 06:00:00 68.222984
230 2031-03-30 12:00:00 68.217010
231 2031-03-30 18:00:00 68.211052
232 2031-03-31 00:00:00 68.205093
233 2031-03-31 06:00:00 68.199120
234 2031-03-31 12:00:00 68.193153
235 2031-02-26 00:00:00 NaN
236 2031-02-26 06:00:00 NaN
237 2031-02-26 12:00:00 NaN
238 2031-02-26 18:00:00 NaN
239 2031-02-27 00:00:00 NaN
240 2031-02-27 06:00:00 NaN
....
369 2031-03-31 12:00:00 NaN
370 2031-03-31 18:00:00 68.258781
371 2031-04-01 00:00:00 67.950096
372 2031-04-01 06:00:00 67.949493
373 2031-04-01 12:00:00 67.949539
374 2031-04-01 18:00:00 67.950241
375 2031-04-02 00:00:00 67.951591
376 2031-04-02 06:00:00 67.953590
377 2031-04-02 12:00:00 67.955589
378 2031-04-02 18:00:00 67.957596
379 2031-04-03 00:00:00 67.959595
380 2031-04-03 06:00:00 67.961601
The real problem I can't fully wrap my head around is that only future.iloc[235]
is an invalid timedelta. future.iloc[236:270]
are still technically correct 6H timesteps, they have just been offset, which causes the duplication. So to fully remove invalid data, I need to identify both the invalid timedelta as well as the valid timedeltas that create the duplicate data.
I have attempted to create a comparison date range with pd.date_range(start=future.iloc[0].time, end=future.iloc[-1].time, freq='6H')
, and iterate through my rows to find faulty values. However, I have not been able to come up with a solution that will actually identify and remove the faulty rows.
Any ideas on how to do this? I assumed pandas would have some built-in functionality for something like this, but haven't been able to find anything substantial that fits my needs.
Bonus: Every check that I have tried seems to take up to minute to run through about 30,000 rows of data. Does this number of rows warrant this time usage iterate through?
python pandas time-series python-xarray
What exactly do you mean by "remove the faulty rows, or null all data that is associated with these rows"? When an inconsistent timedelta is found, you want something like removing rows with dates on the same day ?
– jmiguel
Mar 9 at 4:37
@jmiguel Yes, as well as any data associated with the invalid timedelta. I added some info to my question that will hopefully clarify this further.
– k.mcgee
Mar 9 at 8:21
add a comment |
I looked for an answer for this for a while but haven't been able to find anything, so forgive me if this question has been asked before...
I have some 6-hourly timeseries data future
of future temperature projections for the years 2031-2050. Upon looking at the data, I notice that there is some faulty timedeltas in the dataset starting at future.iloc[234]
:
future.iloc[220:281]
time Temp
220 2031-03-28 00:00:00 68.276657
221 2031-03-28 06:00:00 68.270706
222 2031-03-28 12:00:00 68.264748
223 2031-03-28 18:00:00 68.258781
224 2031-03-29 00:00:00 68.252808
225 2031-03-29 06:00:00 68.246849
226 2031-03-29 12:00:00 68.240883
227 2031-03-29 18:00:00 68.234909
228 2031-03-30 00:00:00 68.228943
229 2031-03-30 06:00:00 68.222984
230 2031-03-30 12:00:00 68.217010
231 2031-03-30 18:00:00 68.211052
232 2031-03-31 00:00:00 68.205093
233 2031-03-31 06:00:00 68.199120
234 2031-03-31 12:00:00 68.193153
235 2031-02-26 00:00:00 68.187195
236 2031-02-26 06:00:00 68.181236
237 2031-02-26 12:00:00 68.175270
238 2031-02-26 18:00:00 68.169304
239 2031-02-27 00:00:00 68.163322
240 2031-02-27 06:00:00 68.169304
....
369 2031-03-31 12:00:00 68.193153
370 2031-03-31 18:00:00 68.258781
371 2031-04-01 00:00:00 67.950096
372 2031-04-01 06:00:00 67.949493
373 2031-04-01 12:00:00 67.949539
374 2031-04-01 18:00:00 67.950241
375 2031-04-02 00:00:00 67.951591
376 2031-04-02 06:00:00 67.953590
377 2031-04-02 12:00:00 67.955589
378 2031-04-02 18:00:00 67.957596
379 2031-04-03 00:00:00 67.959595
380 2031-04-03 06:00:00 67.961601
The dataset continues with the correct timedelta after this blip, but seems to repeat just over a whole month of data (i.e. future.iloc[370] = 2031-03-31 18:00:00
which should be the next timestep after future.iloc[234]
, and continues with valid data from this point on).I know that the data (other than the repeated month) is valid, so I need to try an salvage the data if I can. I have a number of these datasets, so I now fear that they may have faulty time steps in them as well.
My goal is to check for an inconsistent timedelta between two points, and either remove the rows with the invalid timedeltas:
time Temp
220 2031-03-28 00:00:00 68.276657
221 2031-03-28 06:00:00 68.270706
222 2031-03-28 12:00:00 68.264748
223 2031-03-28 18:00:00 68.258781
224 2031-03-29 00:00:00 68.252808
225 2031-03-29 06:00:00 68.246849
226 2031-03-29 12:00:00 68.240883
227 2031-03-29 18:00:00 68.234909
228 2031-03-30 00:00:00 68.228943
229 2031-03-30 06:00:00 68.222984
230 2031-03-30 12:00:00 68.217010
231 2031-03-30 18:00:00 68.211052
232 2031-03-31 00:00:00 68.205093
233 2031-03-31 06:00:00 68.199120
234 2031-03-31 12:00:00 68.193153
235 2031-03-31 18:00:00 68.258781
236 2031-04-01 00:00:00 67.950096
237 2031-04-01 06:00:00 67.949493
238 2031-04-01 12:00:00 67.949539
239 2031-04-01 18:00:00 67.950241
240 2031-04-02 00:00:00 67.951591
241 2031-04-02 06:00:00 67.953590
242 2031-04-02 12:00:00 67.955589
243 2031-04-02 18:00:00 67.957596
244 2031-04-03 00:00:00 67.959595
245 2031-04-03 06:00:00 67.961601
or null all data that is associated with an invalid timedelta:
time Temp
220 2031-03-28 00:00:00 68.276657
221 2031-03-28 06:00:00 68.270706
222 2031-03-28 12:00:00 68.264748
223 2031-03-28 18:00:00 68.258781
224 2031-03-29 00:00:00 68.252808
225 2031-03-29 06:00:00 68.246849
226 2031-03-29 12:00:00 68.240883
227 2031-03-29 18:00:00 68.234909
228 2031-03-30 00:00:00 68.228943
229 2031-03-30 06:00:00 68.222984
230 2031-03-30 12:00:00 68.217010
231 2031-03-30 18:00:00 68.211052
232 2031-03-31 00:00:00 68.205093
233 2031-03-31 06:00:00 68.199120
234 2031-03-31 12:00:00 68.193153
235 2031-02-26 00:00:00 NaN
236 2031-02-26 06:00:00 NaN
237 2031-02-26 12:00:00 NaN
238 2031-02-26 18:00:00 NaN
239 2031-02-27 00:00:00 NaN
240 2031-02-27 06:00:00 NaN
....
369 2031-03-31 12:00:00 NaN
370 2031-03-31 18:00:00 68.258781
371 2031-04-01 00:00:00 67.950096
372 2031-04-01 06:00:00 67.949493
373 2031-04-01 12:00:00 67.949539
374 2031-04-01 18:00:00 67.950241
375 2031-04-02 00:00:00 67.951591
376 2031-04-02 06:00:00 67.953590
377 2031-04-02 12:00:00 67.955589
378 2031-04-02 18:00:00 67.957596
379 2031-04-03 00:00:00 67.959595
380 2031-04-03 06:00:00 67.961601
The real problem I can't fully wrap my head around is that only future.iloc[235]
is an invalid timedelta. future.iloc[236:270]
are still technically correct 6H timesteps, they have just been offset, which causes the duplication. So to fully remove invalid data, I need to identify both the invalid timedelta as well as the valid timedeltas that create the duplicate data.
I have attempted to create a comparison date range with pd.date_range(start=future.iloc[0].time, end=future.iloc[-1].time, freq='6H')
, and iterate through my rows to find faulty values. However, I have not been able to come up with a solution that will actually identify and remove the faulty rows.
Any ideas on how to do this? I assumed pandas would have some built-in functionality for something like this, but haven't been able to find anything substantial that fits my needs.
Bonus: Every check that I have tried seems to take up to minute to run through about 30,000 rows of data. Does this number of rows warrant this time usage iterate through?
python pandas time-series python-xarray
I looked for an answer for this for a while but haven't been able to find anything, so forgive me if this question has been asked before...
I have some 6-hourly timeseries data future
of future temperature projections for the years 2031-2050. Upon looking at the data, I notice that there is some faulty timedeltas in the dataset starting at future.iloc[234]
:
future.iloc[220:281]
time Temp
220 2031-03-28 00:00:00 68.276657
221 2031-03-28 06:00:00 68.270706
222 2031-03-28 12:00:00 68.264748
223 2031-03-28 18:00:00 68.258781
224 2031-03-29 00:00:00 68.252808
225 2031-03-29 06:00:00 68.246849
226 2031-03-29 12:00:00 68.240883
227 2031-03-29 18:00:00 68.234909
228 2031-03-30 00:00:00 68.228943
229 2031-03-30 06:00:00 68.222984
230 2031-03-30 12:00:00 68.217010
231 2031-03-30 18:00:00 68.211052
232 2031-03-31 00:00:00 68.205093
233 2031-03-31 06:00:00 68.199120
234 2031-03-31 12:00:00 68.193153
235 2031-02-26 00:00:00 68.187195
236 2031-02-26 06:00:00 68.181236
237 2031-02-26 12:00:00 68.175270
238 2031-02-26 18:00:00 68.169304
239 2031-02-27 00:00:00 68.163322
240 2031-02-27 06:00:00 68.169304
....
369 2031-03-31 12:00:00 68.193153
370 2031-03-31 18:00:00 68.258781
371 2031-04-01 00:00:00 67.950096
372 2031-04-01 06:00:00 67.949493
373 2031-04-01 12:00:00 67.949539
374 2031-04-01 18:00:00 67.950241
375 2031-04-02 00:00:00 67.951591
376 2031-04-02 06:00:00 67.953590
377 2031-04-02 12:00:00 67.955589
378 2031-04-02 18:00:00 67.957596
379 2031-04-03 00:00:00 67.959595
380 2031-04-03 06:00:00 67.961601
The dataset continues with the correct timedelta after this blip, but seems to repeat just over a whole month of data (i.e. future.iloc[370] = 2031-03-31 18:00:00
which should be the next timestep after future.iloc[234]
, and continues with valid data from this point on).I know that the data (other than the repeated month) is valid, so I need to try an salvage the data if I can. I have a number of these datasets, so I now fear that they may have faulty time steps in them as well.
My goal is to check for an inconsistent timedelta between two points, and either remove the rows with the invalid timedeltas:
time Temp
220 2031-03-28 00:00:00 68.276657
221 2031-03-28 06:00:00 68.270706
222 2031-03-28 12:00:00 68.264748
223 2031-03-28 18:00:00 68.258781
224 2031-03-29 00:00:00 68.252808
225 2031-03-29 06:00:00 68.246849
226 2031-03-29 12:00:00 68.240883
227 2031-03-29 18:00:00 68.234909
228 2031-03-30 00:00:00 68.228943
229 2031-03-30 06:00:00 68.222984
230 2031-03-30 12:00:00 68.217010
231 2031-03-30 18:00:00 68.211052
232 2031-03-31 00:00:00 68.205093
233 2031-03-31 06:00:00 68.199120
234 2031-03-31 12:00:00 68.193153
235 2031-03-31 18:00:00 68.258781
236 2031-04-01 00:00:00 67.950096
237 2031-04-01 06:00:00 67.949493
238 2031-04-01 12:00:00 67.949539
239 2031-04-01 18:00:00 67.950241
240 2031-04-02 00:00:00 67.951591
241 2031-04-02 06:00:00 67.953590
242 2031-04-02 12:00:00 67.955589
243 2031-04-02 18:00:00 67.957596
244 2031-04-03 00:00:00 67.959595
245 2031-04-03 06:00:00 67.961601
or null all data that is associated with an invalid timedelta:
time Temp
220 2031-03-28 00:00:00 68.276657
221 2031-03-28 06:00:00 68.270706
222 2031-03-28 12:00:00 68.264748
223 2031-03-28 18:00:00 68.258781
224 2031-03-29 00:00:00 68.252808
225 2031-03-29 06:00:00 68.246849
226 2031-03-29 12:00:00 68.240883
227 2031-03-29 18:00:00 68.234909
228 2031-03-30 00:00:00 68.228943
229 2031-03-30 06:00:00 68.222984
230 2031-03-30 12:00:00 68.217010
231 2031-03-30 18:00:00 68.211052
232 2031-03-31 00:00:00 68.205093
233 2031-03-31 06:00:00 68.199120
234 2031-03-31 12:00:00 68.193153
235 2031-02-26 00:00:00 NaN
236 2031-02-26 06:00:00 NaN
237 2031-02-26 12:00:00 NaN
238 2031-02-26 18:00:00 NaN
239 2031-02-27 00:00:00 NaN
240 2031-02-27 06:00:00 NaN
....
369 2031-03-31 12:00:00 NaN
370 2031-03-31 18:00:00 68.258781
371 2031-04-01 00:00:00 67.950096
372 2031-04-01 06:00:00 67.949493
373 2031-04-01 12:00:00 67.949539
374 2031-04-01 18:00:00 67.950241
375 2031-04-02 00:00:00 67.951591
376 2031-04-02 06:00:00 67.953590
377 2031-04-02 12:00:00 67.955589
378 2031-04-02 18:00:00 67.957596
379 2031-04-03 00:00:00 67.959595
380 2031-04-03 06:00:00 67.961601
The real problem I can't fully wrap my head around is that only future.iloc[235]
is an invalid timedelta. future.iloc[236:270]
are still technically correct 6H timesteps, they have just been offset, which causes the duplication. So to fully remove invalid data, I need to identify both the invalid timedelta as well as the valid timedeltas that create the duplicate data.
I have attempted to create a comparison date range with pd.date_range(start=future.iloc[0].time, end=future.iloc[-1].time, freq='6H')
, and iterate through my rows to find faulty values. However, I have not been able to come up with a solution that will actually identify and remove the faulty rows.
Any ideas on how to do this? I assumed pandas would have some built-in functionality for something like this, but haven't been able to find anything substantial that fits my needs.
Bonus: Every check that I have tried seems to take up to minute to run through about 30,000 rows of data. Does this number of rows warrant this time usage iterate through?
python pandas time-series python-xarray
python pandas time-series python-xarray
edited Mar 9 at 8:20
k.mcgee
asked Mar 9 at 2:28
k.mcgeek.mcgee
565
565
What exactly do you mean by "remove the faulty rows, or null all data that is associated with these rows"? When an inconsistent timedelta is found, you want something like removing rows with dates on the same day ?
– jmiguel
Mar 9 at 4:37
@jmiguel Yes, as well as any data associated with the invalid timedelta. I added some info to my question that will hopefully clarify this further.
– k.mcgee
Mar 9 at 8:21
add a comment |
What exactly do you mean by "remove the faulty rows, or null all data that is associated with these rows"? When an inconsistent timedelta is found, you want something like removing rows with dates on the same day ?
– jmiguel
Mar 9 at 4:37
@jmiguel Yes, as well as any data associated with the invalid timedelta. I added some info to my question that will hopefully clarify this further.
– k.mcgee
Mar 9 at 8:21
What exactly do you mean by "remove the faulty rows, or null all data that is associated with these rows"? When an inconsistent timedelta is found, you want something like removing rows with dates on the same day ?
– jmiguel
Mar 9 at 4:37
What exactly do you mean by "remove the faulty rows, or null all data that is associated with these rows"? When an inconsistent timedelta is found, you want something like removing rows with dates on the same day ?
– jmiguel
Mar 9 at 4:37
@jmiguel Yes, as well as any data associated with the invalid timedelta. I added some info to my question that will hopefully clarify this further.
– k.mcgee
Mar 9 at 8:21
@jmiguel Yes, as well as any data associated with the invalid timedelta. I added some info to my question that will hopefully clarify this further.
– k.mcgee
Mar 9 at 8:21
add a comment |
1 Answer
1
active
oldest
votes
to identify the errors maybe to do this sort of test:
1) you group by day
2) you trap the group with number items > 0 and < 4
3) you have the list of errors, you could drop the corresponding rows
errorlist=[]
def f(g):
if g.shape[0] > 0 and g.shape[0] < 4:
errorlist.append(g.index[0])
df.set_index('time').groupby(pd.Grouper(freq='D')).apply(f)
print(errorlist)
add a comment |
Your Answer
StackExchange.ifUsing("editor", function ()
StackExchange.using("externalEditor", function ()
StackExchange.using("snippets", function ()
StackExchange.snippets.init();
);
);
, "code-snippets");
StackExchange.ready(function()
var channelOptions =
tags: "".split(" "),
id: "1"
;
initTagRenderer("".split(" "), "".split(" "), channelOptions);
StackExchange.using("externalEditor", function()
// Have to fire editor after snippets, if snippets enabled
if (StackExchange.settings.snippets.snippetsEnabled)
StackExchange.using("snippets", function()
createEditor();
);
else
createEditor();
);
function createEditor()
StackExchange.prepareEditor(
heartbeatType: 'answer',
autoActivateHeartbeat: false,
convertImagesToLinks: true,
noModals: true,
showLowRepImageUploadWarning: true,
reputationToPostImages: 10,
bindNavPrevention: true,
postfix: "",
imageUploader:
brandingHtml: "Powered by u003ca class="icon-imgur-white" href="https://imgur.com/"u003eu003c/au003e",
contentPolicyHtml: "User contributions licensed under u003ca href="https://creativecommons.org/licenses/by-sa/3.0/"u003ecc by-sa 3.0 with attribution requiredu003c/au003e u003ca href="https://stackoverflow.com/legal/content-policy"u003e(content policy)u003c/au003e",
allowUrls: true
,
onDemand: true,
discardSelector: ".discard-answer"
,immediatelyShowMarkdownHelp:true
);
);
Sign up or log in
StackExchange.ready(function ()
StackExchange.helpers.onClickDraftSave('#login-link');
);
Sign up using Google
Sign up using Facebook
Sign up using Email and Password
Post as a guest
Required, but never shown
StackExchange.ready(
function ()
StackExchange.openid.initPostLogin('.new-post-login', 'https%3a%2f%2fstackoverflow.com%2fquestions%2f55073437%2fidentifying-and-removing-invalid-time-steps-in-a-pandas-time-series%23new-answer', 'question_page');
);
Post as a guest
Required, but never shown
1 Answer
1
active
oldest
votes
1 Answer
1
active
oldest
votes
active
oldest
votes
active
oldest
votes
to identify the errors maybe to do this sort of test:
1) you group by day
2) you trap the group with number items > 0 and < 4
3) you have the list of errors, you could drop the corresponding rows
errorlist=[]
def f(g):
if g.shape[0] > 0 and g.shape[0] < 4:
errorlist.append(g.index[0])
df.set_index('time').groupby(pd.Grouper(freq='D')).apply(f)
print(errorlist)
add a comment |
to identify the errors maybe to do this sort of test:
1) you group by day
2) you trap the group with number items > 0 and < 4
3) you have the list of errors, you could drop the corresponding rows
errorlist=[]
def f(g):
if g.shape[0] > 0 and g.shape[0] < 4:
errorlist.append(g.index[0])
df.set_index('time').groupby(pd.Grouper(freq='D')).apply(f)
print(errorlist)
add a comment |
to identify the errors maybe to do this sort of test:
1) you group by day
2) you trap the group with number items > 0 and < 4
3) you have the list of errors, you could drop the corresponding rows
errorlist=[]
def f(g):
if g.shape[0] > 0 and g.shape[0] < 4:
errorlist.append(g.index[0])
df.set_index('time').groupby(pd.Grouper(freq='D')).apply(f)
print(errorlist)
to identify the errors maybe to do this sort of test:
1) you group by day
2) you trap the group with number items > 0 and < 4
3) you have the list of errors, you could drop the corresponding rows
errorlist=[]
def f(g):
if g.shape[0] > 0 and g.shape[0] < 4:
errorlist.append(g.index[0])
df.set_index('time').groupby(pd.Grouper(freq='D')).apply(f)
print(errorlist)
answered Mar 9 at 5:21
FrenchyFrenchy
2,5662518
2,5662518
add a comment |
add a comment |
Thanks for contributing an answer to Stack Overflow!
- Please be sure to answer the question. Provide details and share your research!
But avoid …
- Asking for help, clarification, or responding to other answers.
- Making statements based on opinion; back them up with references or personal experience.
To learn more, see our tips on writing great answers.
Sign up or log in
StackExchange.ready(function ()
StackExchange.helpers.onClickDraftSave('#login-link');
);
Sign up using Google
Sign up using Facebook
Sign up using Email and Password
Post as a guest
Required, but never shown
StackExchange.ready(
function ()
StackExchange.openid.initPostLogin('.new-post-login', 'https%3a%2f%2fstackoverflow.com%2fquestions%2f55073437%2fidentifying-and-removing-invalid-time-steps-in-a-pandas-time-series%23new-answer', 'question_page');
);
Post as a guest
Required, but never shown
Sign up or log in
StackExchange.ready(function ()
StackExchange.helpers.onClickDraftSave('#login-link');
);
Sign up using Google
Sign up using Facebook
Sign up using Email and Password
Post as a guest
Required, but never shown
Sign up or log in
StackExchange.ready(function ()
StackExchange.helpers.onClickDraftSave('#login-link');
);
Sign up using Google
Sign up using Facebook
Sign up using Email and Password
Post as a guest
Required, but never shown
Sign up or log in
StackExchange.ready(function ()
StackExchange.helpers.onClickDraftSave('#login-link');
);
Sign up using Google
Sign up using Facebook
Sign up using Email and Password
Sign up using Google
Sign up using Facebook
Sign up using Email and Password
Post as a guest
Required, but never shown
Required, but never shown
Required, but never shown
Required, but never shown
Required, but never shown
Required, but never shown
Required, but never shown
Required, but never shown
Required, but never shown
2Ibz5RkdAbxAtC2Od GcYExn
What exactly do you mean by "remove the faulty rows, or null all data that is associated with these rows"? When an inconsistent timedelta is found, you want something like removing rows with dates on the same day ?
– jmiguel
Mar 9 at 4:37
@jmiguel Yes, as well as any data associated with the invalid timedelta. I added some info to my question that will hopefully clarify this further.
– k.mcgee
Mar 9 at 8:21